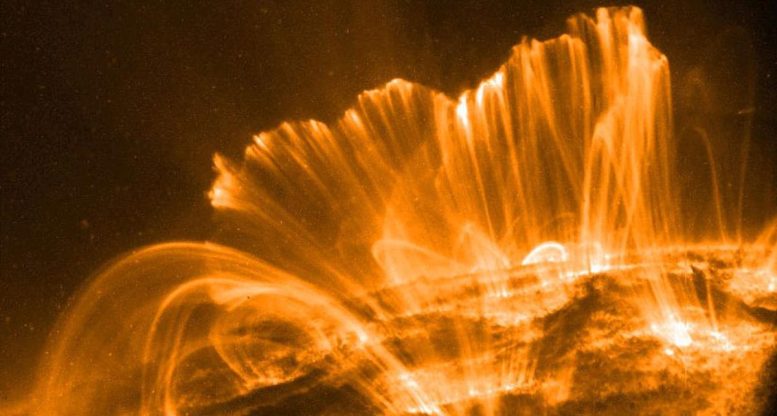
Naukowcy z Uniwersytetu Hawajskiego wykorzystują moc największego na świecie teleskopu słonecznego i zaawansowanej sztucznej inteligencji, aby zmienić nasze rozumienie Słońca.
Ich projekt ma na celu szybką analizę ogromnych zbiorów danych, aby lepiej przewidywać zjawiska słoneczne, takie jak burze słoneczne, które mogą prowadzić do postępów w[{” attribute=”” tabindex=”0″ role=”link”>accuracy and speed of solar research.
Revolutionary Solar Research
Astronomers and computer scientists at the University of Hawaiʻi Institute for Astronomy (IfA) are conducting groundbreaking research that could revolutionize our understanding of the Sun.
As part of the “SPIn4D” project, the team is merging advanced solar astronomy with cutting-edge computer science to process data collected by the world’s largest ground-based solar telescope, located atop Haleakalā in Maui.
Their work, recently published in the Astrophysical Journal, centers on developing deep learning models capable of rapidly analyzing the massive datasets generated by the NSF’s Daniel K. Inouye Solar Telescope. These efforts aim to maximize the telescope’s capabilities, paving the way for significant advances in the speed, accuracy, and depth of solar data analysis.
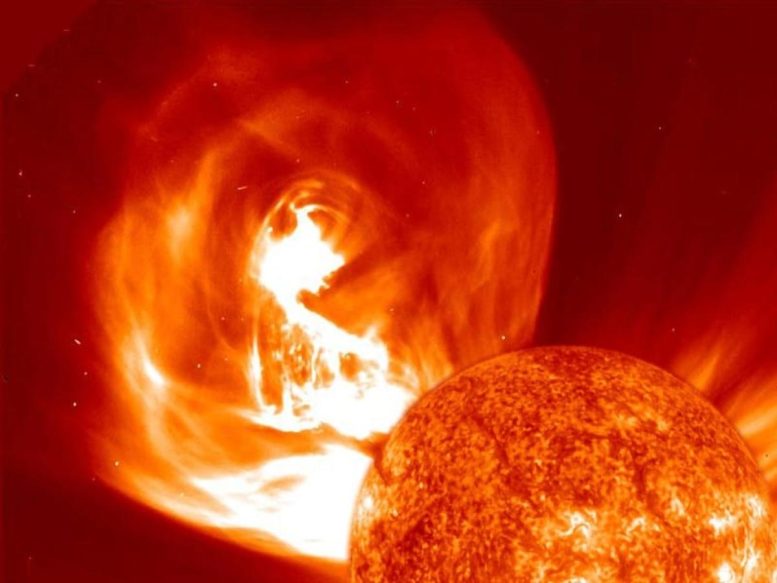
The Importance of Understanding Solar Storms
“Large solar storms are responsible for stunning auroras, but can also pose risks to satellites, radio communications, and power grids. A better understanding of their birthplace, the solar atmosphere, is extremely important,” said Kai Yang, an IfA postdoctoral researcher who led the work. “We used state-of-the-art simulations to mimic what the Inouye will see. Combining these data with machine learning offers an invaluable opportunity to explore the three-dimensional solar atmosphere in near real-time.”
The Inouye Solar Telescope, operated by the NSF National Solar Observatory (NSO), is by far the world’s most powerful solar telescope, and stands on the 10,000-foot summit of Maui’s Haleakalā, which translates to “the house of the Sun.” The telescope’s instruments are designed to measure the Sun’s magnetic field using polarized light, and the SPIn4D project was designed specifically to use this data, which is only available from the solar telescope’s instrumentation suite.
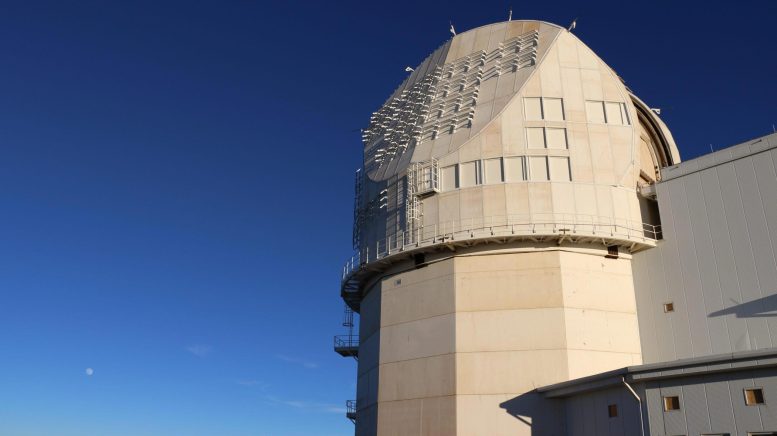
AI-Driven Analysis of the Solar Atmosphere
The team of scientists from NSO and High Altitude Observatory (HAO) utilizes deep neural networks to estimate the physical properties of the solar photosphere from the Inouye Solar Telescope’s high-resolution observations. This method promises to significantly speed up the analysis of the massive data volumes produced by the solar telescope, which can reach tens of terabytes per day.
“Machine learning is very good at providing fast approximations to expensive computations. In this case, the model will enable astronomers to visualize the Sun’s atmosphere in real-time, rather than waiting hours to achieve the same accuracy,” said co-author Peter Sadowski, an associate professor at the UH Mānoa information and computer sciences department.
Opracowywanie narzędzi AI do danych słonecznych
Aby wytrenować modele sztucznej inteligencji, zespół stworzył obszerny zbiór danych obejmujący symulowane obserwacje Słońca. Wykorzystując ponad 10 milionów godzin procesora na superkomputerze Cheyenne należącym do NSF, utworzyli 120 terabajtów danych naśladujących obserwacje z Teleskopu Słonecznego Inouye w niezwykle wysokiej rozdzielczości.
Zespół udostępnił już publicznie 13-terabajtowy podzbiór swoich danych wraz ze szczegółowym samouczkiem. Planują udostępnić swoje w pełni wyszkolone modele głębokiego uczenia się jako narzędzie społecznościowe do analizy obserwacji Teleskopu Słonecznego Inouye.
Odniesienie: „Inwersja spektropolarymetryczna w czterech wymiarach z głębokim uczeniem się (SPIn4D). I. Przegląd, modelowanie magnetohydrodynamiczne i synteza profilu Stokesa” autorstwa Kai E. Yang, Lucas A. Tarr, Matthias Rempel, S. Curt Dodds, Sarah A. Jaeggli, Peter Sadowski, Thomas A. Schad, Ian Cunnyngham, Jiayi Liu, Yannik Glaser i Xudong Sun, 25 listopada 2024 r., Dziennik astrofizyczny.
DOI: 10.3847/1538-4357/ad865b
Badania te są wspierane przez grant w wysokości 669 000 dolarów z programu NSF Astronomy and Astrophysics Grants. W skład zespołu naukowców wchodzą Yang, Xudong Sun, Ian Cunningham, Jiayi Liu, Curt Dodds (UH IfA), Sadowski, Yannik Glaser (UH Mānoa ICS) oraz Sarah Jaeggli, Tom Schad, Lucas Tarr (NSO) i Matthias Rempel (HAO).