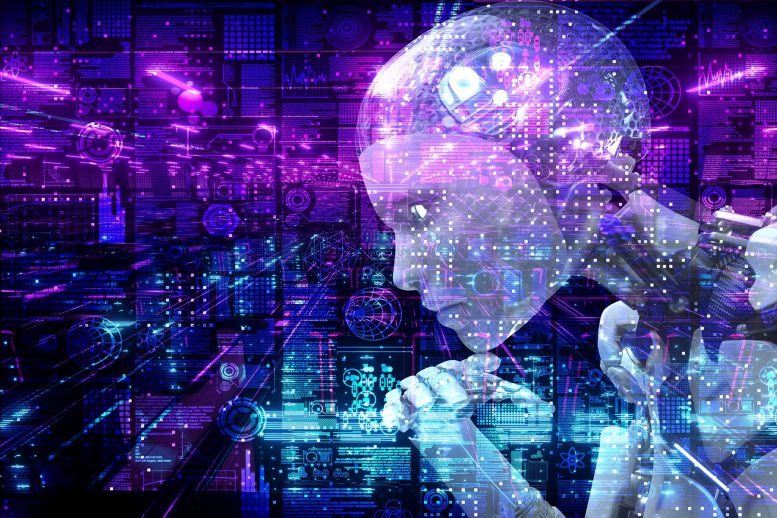
Naukowcy z laboratorium Cold Spring Harbor opracowali algorytm sztucznej inteligencji inspirowany wydajnością genomu, osiągając niezwykłą kompresję danych i wydajność zadań.
W pewnym sensie każdy z nas zaczyna życie gotowy do działania. Wiele zwierząt dokonuje niesamowitych wyczynów wkrótce po urodzeniu. Pająki przędą sieci. Wieloryby pływają. Ale skąd biorą się te wrodzone zdolności? Oczywiście mózg odgrywa kluczową rolę, ponieważ zawiera biliony połączeń neuronowych potrzebnych do kontrolowania złożonych zachowań.
Jednak w genomie jest miejsce tylko na niewielką część tej informacji. Ten paradoks zdumiewa naukowców od dziesięcioleci. Teraz profesorowie z Cold Spring Harbor Laboratory (CSHL) Anthony Zador i Alexei Koulakov opracowali potencjalne rozwiązanie wykorzystujące[{” attribute=”” tabindex=”0″ role=”link”>artificial intelligence.
When Zador first encounters this problem, he puts a new spin on it. “What if the genome’s limited capacity is the very thing that makes us so smart?” he wonders. “What if it’s a feature, not a bug?” In other words, maybe we can act intelligently and learn quickly because the genome’s limits force us to adapt. This is a big, bold idea—tough to demonstrate. After all, we can’t stretch lab experiments across billions of years of evolution. That’s where the idea of the genomic bottleneck algorithm emerges.
Using AI to Mimic Evolutionary Efficiency
In AI, generations don’t span decades. New models are born with the push of a button. Zador, Koulakov, and CSHL postdocs Divyansha Lachi and Sergey Shuvaev set out to develop a computer algorithm that folds heaps of data into a neat package—much like our genome might compress the information needed to form functional brain circuits. They then test this algorithm against AI networks that undergo multiple training rounds. Amazingly, they find the new, untrained algorithm performs tasks like image recognition almost as effectively as state-of-the-art AI. Their algorithm even holds its own in video games like Space Invaders. It’s as if it innately understands how to play.
Does this mean AI will soon replicate our natural abilities? “We haven’t reached that level,” says Koulakov. “The brain’s cortical architecture can fit about 280 terabytes of information—32 years of high-definition video. Our genomes accommodate about one hour. This implies a 400,000-fold compression technology cannot yet match.”
Nevertheless, the algorithm allows for compression levels thus far unseen in AI. That feature could have impressive uses in tech. Shuvaev, the study’s lead author, explains: “For example, if you wanted to run a large language model on a cell phone, one way [the algorithm] można zastosować, to rozkładanie modelu warstwa po warstwie na sprzęcie.”
Takie aplikacje mogą oznaczać bardziej rozwiniętą sztuczną inteligencję z krótszym czasem działania. I pomyśleć, że ewolucja potrzebowała zaledwie 3,5 miliarda lat, aby tu dotrzeć.
Odniesienie: „Kodowanie wrodzonych zdolności przez wąskie gardło genomu” autorstwa Sergeya Shuvaeva, Divyansha Lachi, Alexei Koulakov i Anthony Zador, 12 września 2024 r., Postępowanie Narodowej Akademii Nauk.
DOI: 10.1073/pnas.2409160121
Badanie zostało sfinansowane przez Deep Valley Labs, Fundację G. Harolda i Leili Y. Mathers oraz Schmidt Futures.